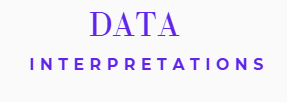
System GMM: Dynamic Panel Data Analysis
System Generalized Method of Moments (System GMM) is a powerful econometric technique used for estimating dynamic panel data models. It addresses several issues that arise when dealing with panel data, such as endogeneity, unobserved heterogeneity, and autocorrelation. System GMM, developed by Arellano and Bover (1995) and Blundell and Bond (1998), is particularly useful for models where the dependent variable’s past values influence its current values, making it a popular tool in empirical economics, finance, and other social sciences.
- Understanding Panel Data
Panel data, also known as longitudinal data, consists of observations on multiple entities (such as individuals, firms, or countries) over time. A typical panel dataset contains both time-series and cross-sectional dimensions. Panel data models offer several advantages over pure time series or cross-sectional models because they allow the researcher to control for individual-specific effects and capture both the temporal dynamics and individual heterogeneity.
- The Need for System GMM
In dynamic panel data models, lagged values of the dependent variable are often included as explanatory variables to account for inertia or adjustment processes. However, this can create a problem of endogeneity because the lagged dependent variable is correlated with the error term. Endogeneity also arises from the presence of time-invariant unobserved factors (e.g., culture or organizational structure) that affect the dependent variable and may be correlated with the independent variables.
Standard estimators like Ordinary Least Squares (OLS) or Fixed Effects (FE) regressions are inadequate in these cases. OLS leads to biased and inconsistent estimates due to the correlation between the lagged dependent variable and the error term. FE methods, while they control for unobserved heterogeneity, do not solve the endogeneity issue and often perform poorly in dynamic settings.
To overcome these challenges, econometricians have developed methods like the Generalized Method of Moments (GMM), with System GMM being one of the most efficient solutions for dynamic panel data models.
- How System GMM Works
System GMM combines two sets of moment conditions derived from the data: one from the model in levels and one from the model in differences. These two sets of moment conditions help in addressing the endogeneity problem effectively by using internal instruments—typically lagged values of the endogenous variables.
3.1 First-Difference GMM
The initial approach developed by Arellano and Bond (1991) is called Difference GMM. It removes the individual-specific effects by differencing the model, and it uses lagged values of the endogenous variables as instruments in the first-differenced equations. This method solves the endogeneity problem but has some weaknesses. For example, if the variables are highly persistent, the lagged values may not be strong instruments, leading to weak instrument problems and poor finite sample performance.
3.2 System GMM
System GMM builds on the Difference GMM by augmenting it with additional moment conditions. Specifically, it uses both the equations in first differences and the equations in levels, thus leading to a “system” of equations. For the first-difference equation, lagged levels are used as instruments, while for the level equation, lagged differences are used as instruments. This dual approach allows System GMM to improve efficiency and perform better in the presence of weak instruments or highly persistent variables.
- Assumptions of System GMM
System GMM relies on certain assumptions to produce consistent estimates:
- Instrument Validity: The instruments must be valid, meaning they should be exogenous and not correlated with the error term. The validity of instruments can be tested using the Hansen test (also known as the J-test).
- No Second-Order Autocorrelation: System GMM assumes that there is no second-order autocorrelation in the differenced errors, which can be checked using the Arellano-Bond test.
- Stationarity: It assumes that the variables in the model are stationary or exhibit weakly exogenous behavior over time.
- Advantages of System GMM
- Endogeneity Control: One of the primary advantages of System GMM is its ability to control for endogeneity by using internal instruments, thus providing consistent and efficient estimates.
- Unobserved Heterogeneity: System GMM accounts for time-invariant individual-specific effects, which may otherwise bias the estimates.
- Efficiency in Dynamic Models: System GMM performs well in dynamic settings, especially when the dependent variable’s past values influence its current values.
- Suitable for Short Panels: System GMM is particularly useful in situations where the number of time periods (T) is small, but the number of individuals or units (N) is large, which is a common feature of many panel datasets in economics and finance.
- Limitations and Challenges
- Instrument Proliferation: One common issue with System GMM is the proliferation of instruments, which can lead to overfitting and a failure of the Hansen test for instrument validity. Researchers must use caution when selecting the number of lags to be used as instruments and sometimes employ techniques like collapsing the instrument matrix.
- Assumption Sensitivity: The results from System GMM are sensitive to the underlying assumptions of instrument validity and no second-order autocorrelation. Violations of these assumptions can lead to biased estimates.
- Finite Sample Bias: Although System GMM performs better than traditional methods in many cases, it can still suffer from finite sample bias in small datasets.
- Applications of System GMM
System GMM is widely used in empirical research, particularly in fields such as macroeconomics, finance, labor economics, and development economics. Some common applications include:
- Economic Growth Models: Researchers often use System GMM to estimate growth regressions where past economic growth influences future growth rates.
- Corporate Finance: In studies of corporate financial decisions (e.g., capital structure or investment), System GMM helps account for dynamic relationships and endogeneity.
- Labor Market Studies: It is used to model wage dynamics and employment behavior where past wages or employment status influence current outcomes.
System GMM is a highly valuable technique for addressing the challenges posed by dynamic panel data models. By leveraging the power of internal instruments and combining equations in levels and differences, System GMM provides more reliable and efficient estimates than traditional methods like OLS or FE. However, like any econometric tool, it requires careful application, particularly in the choice of instruments and in verifying the underlying assumptions. When applied correctly, System GMM can yield robust insights into complex dynamic relationships in economics, finance, and other social sciences.