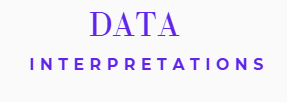
Data Science, Data Analytics, and Their Applications
In the rapidly evolving technological landscape, data has become a crucial asset for organizations and individuals alike. As a result, data science and data analytics have emerged as essential fields for deriving meaningful insights from vast amounts of information. These disciplines are transforming industries by enabling data-driven decision-making and innovation. In this essay, we will explore the concepts of data science and data analytics, their differences, and their wide-ranging applications in various fields.
Data Science: An Overview
Data science is an interdisciplinary field that focuses on extracting knowledge and insights from structured and unstructured data. It combines elements from mathematics, statistics, computer science, and domain expertise to analyze data and uncover patterns. Data scientists use various techniques such as machine learning, artificial intelligence (AI), statistical modeling, and data visualization to make predictions, automate processes, and solve complex problems.
At its core, data science involves the following steps:
- Data Collection: Gathering raw data from various sources, including databases, sensors, social media, and more.
- Data Cleaning: Removing inconsistencies, errors, and missing values from the dataset to ensure accuracy.
- Data Exploration: Analyzing data to understand its structure and uncover hidden patterns.
- Model Building: Using algorithms and statistical models to make predictions or classify data.
- Model Evaluation: Testing the model’s accuracy and performance against unseen data.
- Deployment: Integrating the model into real-world applications for decision-making or automation.
Data science has the power to make sense of complex datasets and provide actionable insights, making it an invaluable tool in various industries.
Data Analytics: A Closer Look
Data analytics is a subset of data science that focuses on analyzing data to answer specific questions, generate reports, and support decision-making processes. While data science is broader and often deals with predictive or prescriptive analysis, data analytics is typically concerned with descriptive analysis—understanding historical data to identify trends and patterns.
Data analytics involves four key types:
- Descriptive Analytics: Provides insights into what happened by summarizing historical data.
- Diagnostic Analytics: Explains why something happened by identifying correlations and patterns.
- Predictive Analytics: Forecasts future trends based on historical data and modeling techniques.
- Prescriptive Analytics: Recommends actions based on the analysis of potential outcomes.
While data analytics is primarily focused on analyzing and interpreting past data, it is instrumental in helping businesses optimize operations, identify opportunities, and make informed decisions.
Applications of Data Science and Data Analytics
Data science and data analytics are transforming various sectors by providing organizations with the tools to harness the power of data. Below are some of the key industries where these fields have made significant contributions:
- Healthcare:
- Data science and analytics are used to improve patient care, predict disease outbreaks, and develop personalized treatment plans. For example, predictive analytics can forecast patient readmission risks, helping healthcare providers take proactive measures.
- AI-powered data science models can assist in diagnosing diseases by analyzing medical imaging, pathology reports, and genetic data.
- Finance:
- In the finance sector, data analytics is employed to detect fraudulent activities, assess credit risks, and optimize investment portfolios. Predictive models can help identify trends in stock markets and suggest the best investment strategies.
- Data science models can analyze customer transaction histories to tailor personalized financial products and services, enhancing customer satisfaction.
- Retail and E-Commerce:
- Retailers leverage data analytics to understand customer preferences, optimize supply chains, and forecast demand. Through recommendation engines, e-commerce platforms use data science to suggest products to customers, driving sales and enhancing the shopping experience.
- Data analytics allows businesses to manage inventory more effectively, reducing waste and improving profitability.
- Marketing and Advertising:
- Data analytics helps marketers track customer behavior, evaluate the success of campaigns, and allocate budgets more efficiently. By analyzing customer data, marketers can create targeted advertising strategies to reach the right audience at the right time.
- Predictive analytics in marketing helps identify potential leads, enabling companies to engage with prospects who are most likely to convert into customers.
- Manufacturing:
- In the manufacturing industry, data science and analytics are used for predictive maintenance, enabling companies to identify equipment failures before they occur. This reduces downtime and maintenance costs.
- Data analytics also helps manufacturers optimize production processes, improve quality control, and enhance overall efficiency by analyzing data from sensors and production lines.
- Transportation and Logistics:
- In the transportation industry, data analytics plays a crucial role in route optimization, fuel efficiency, and fleet management. Predictive models help companies anticipate traffic patterns and adjust routes to minimize delays and costs.
- Logistics companies leverage data science to streamline supply chains, monitor shipments, and improve delivery times by analyzing real-time data from GPS systems, weather conditions, and historical records.
- Sports Analytics:
- Sports teams and athletes use data analytics to enhance performance, scout talent, and develop game strategies. By analyzing player statistics, injury records, and performance metrics, coaches can make data-driven decisions to gain a competitive advantage.
- Predictive models can also be used to forecast game outcomes and assess the impact of various factors on player performance, such as fatigue, weather, or travel schedules.
- Energy Sector:
- In the energy industry, data science is used to optimize energy production and consumption. Predictive analytics models help forecast energy demand, enabling power companies to allocate resources efficiently.
- Renewable energy companies use data science to analyze weather patterns, predict solar or wind energy output, and optimize power generation from renewable sources.
Data science and data analytics have revolutionized the way organizations operate, offering unprecedented opportunities for innovation, optimization, and competitive advantage. By leveraging the power of data, businesses can make informed decisions, enhance efficiency, and improve customer experiences. As technology continues to advance, the fields of data science and data analytics will play an even more critical role in shaping the future of industries across the globe.
Whether in healthcare, finance, retail, or transportation, the applications of data science and data analytics are vast, and their impact is bound to grow as more organizations recognize the value of data-driven insights.