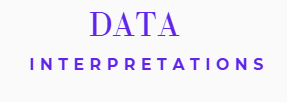
Hypothesis Testing
Hypothesis testing is a fundamental statistical tool used to make inferences about populations based on sample data. It is widely applied in various fields, including scientific research, economics, business, medicine, and social sciences. Hypothesis testing allows researchers to assess the evidence against a null hypothesis by determining whether observed data is consistent with the assumed population characteristics.
- Key Concepts in Hypothesis Testing
At the core of hypothesis testing are two competing hypotheses:
- Null Hypothesis (H₀): The statement that there is no effect or no difference, which the researcher seeks to test. It assumes that any observed effect is due to random chance.
- Alternative Hypothesis (H₁ or Ha): The statement that contradicts the null hypothesis. It suggests that there is an effect, difference, or relationship between variables.
For example, in a clinical trial testing the effectiveness of a new drug, the null hypothesis might state that the drug has no effect on patients, while the alternative hypothesis suggests that the drug has a positive effect.
- Steps in Hypothesis Testing
The process of hypothesis testing typically follows these steps:
- a) State the Hypotheses
The first step is to formulate both the null and alternative hypotheses. For example:
- Null hypothesis (H₀): The new teaching method has no effect on students’ test scores.
- Alternative hypothesis (H₁): The new teaching method improves students’ test scores.
- b) Select the Significance Level (α)
The significance level (α) is the probability of rejecting the null hypothesis when it is true, commonly set at 0.05 (5%). This represents the researcher’s willingness to accept a 5% risk of a Type I error (false positive).
- c) Collect and Summarize the Data
Researchers gather sample data and calculate a test statistic. Common tests include the z-test, t-test, chi-square test, and ANOVA, depending on the nature of the data and hypothesis. The test statistic provides a measure to compare the sample data against the null hypothesis.
- d) Compute the p-value
The p-value represents the probability of observing the sample data, or more extreme values, assuming the null hypothesis is true. A small p-value (typically less than α) suggests strong evidence against H₀.
- e) Make a Decision
Based on the p-value:
- If the p-value is less than α, the null hypothesis is rejected, suggesting that the alternative hypothesis may be true.
- If the p-value is greater than or equal to α, the null hypothesis cannot be rejected, meaning there is not enough evidence to support H₁.
- f) Interpret the Results
Once the decision is made, researchers interpret the results in the context of the study. Rejecting the null hypothesis doesn’t necessarily mean that the alternative hypothesis is definitively true, but rather that the data provides strong evidence against H₀.
- Errors in Hypothesis Testing
Two types of errors can occur in hypothesis testing:
- Type I Error (False Positive): Occurs when the null hypothesis is rejected when it is actually true. The probability of making a Type I error is equal to the significance level (α). A smaller α reduces the chance of this error but increases the chance of a Type II error.
- Type II Error (False Negative): Occurs when the null hypothesis is not rejected when it is actually false. The probability of a Type II error is denoted by β, and 1 – β is the power of the test, which represents the test’s ability to detect an effect when one exists.
- Types of Hypothesis Tests
There are various hypothesis tests, each suited to different data types and research questions:
- One-tailed vs. Two-tailed Tests: A one-tailed test examines if the effect occurs in one specific direction, while a two-tailed test checks for an effect in either direction.
- Parametric vs. Non-parametric Tests: Parametric tests, like the t-test or z-test, rely on assumptions about the population distribution, while non-parametric tests, like the Wilcoxon signed-rank test, do not assume a specific distribution.
- Applications of Hypothesis Testing
Hypothesis testing has a wide range of applications across multiple disciplines:
- In medicine, it is used to test the effectiveness of new treatments and drugs.
- In business, it can assess whether a change in strategy affects sales or customer satisfaction.
- In psychology, it helps determine if a psychological intervention influences behavior.
- In economics, hypothesis testing is used to evaluate economic models and policies.
For example, suppose a company wants to know if its new advertising campaign increased sales. They would set up a hypothesis test with the null hypothesis stating that the campaign had no effect on sales, and the alternative hypothesis suggesting that sales improved.
- Limitations of Hypothesis Testing
While hypothesis testing is a powerful tool, it has some limitations:
- Misinterpretation of p-values: Many researchers mistakenly interpret p-values as the probability that the null hypothesis is true, which is not correct. The p-value only indicates the likelihood of observing the data given that H₀ is true.
- Dependence on sample size: Larger sample sizes can make even trivial differences statistically significant, while smaller sample sizes may fail to detect meaningful effects.
- Subjectivity in α selection: The significance level is chosen arbitrarily, which can influence the outcome of the test.
Hypothesis testing is a critical method in the realm of statistical inference. It provides a structured way to evaluate claims or hypotheses about a population based on sample data. However, careful consideration must be given to the choice of significance level, the type of test used, and the interpretation of results. Despite its limitations, hypothesis testing remains a cornerstone of empirical research and decision-making across many fields.