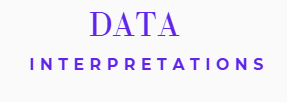
Criteria for Data Strategies
In today’s data-driven world, a manufacturing company must leverage data not only as a supporting function but as a strategic asset that drives innovation, efficiency, and competitive advantage. A sound data strategy forms the backbone of digital transformation, aligning data practices with business goals. The following criteria are critical in formulating an effective data strategy in such an enterprise.
- Business Alignment and Value Realization
The primary criterion is that the data strategy must align with the company’s overarching business objectives. Whether it’s improving operational efficiency, enhancing product quality, reducing supply chain disruptions, or accelerating time-to-market, the strategy should prioritize use cases that deliver tangible business value. Metrics for success should be well-defined and directly tied to KPIs such as production uptime, inventory turnover, or customer satisfaction.
- Data Governance and Compliance
Given the scale and complexity of operations in a manufacturer, establishing strong data governance is essential. This includes setting up clear policies for data ownership, data quality, access controls, and compliance with regulations such as GDPR, CCPA, and industry-specific standards (e.g., ISO 9001 for quality management). A governance framework must be scalable and auditable, fostering trust and accountability across all data stakeholders.
- Scalable Data Architecture
Manufacturing enterprises deal with massive volumes of structured and unstructured data generated from IoT sensors, ERP systems, CAD files, PLM systems, and external sources. A scalable and flexible data architecture—often based on cloud-native platforms—should support real-time ingestion, processing, storage, and analytics. Technologies such as data lakes, data meshes, and hybrid-cloud infrastructure are essential enablers for such scalability.
- Data Integration Across the Value Chain
An effective strategy must ensure seamless integration of data across the entire manufacturing value chain—from product design and raw material sourcing to production, logistics, and customer support. Integration across disparate systems (ERP, MES, CRM, SCM) enables end-to-end visibility and intelligent decision-making. Interoperability standards such as OPC UA or APIs facilitate this integration.
- Advanced Analytics and AI Readiness
Data strategies should incorporate mechanisms for advanced analytics, including predictive maintenance, demand forecasting, quality inspection, and digital twins. AI and machine learning models require curated, high-quality training datasets. Therefore, the strategy must address not only the availability of data but also the preparation of data pipelines, model governance, and continuous model monitoring.
- Cybersecurity and Data Privacy
Manufacturers are increasingly targeted by cyber threats due to the convergence of IT and OT environments. The data strategy must embed cybersecurity measures such as encryption, network segmentation, zero-trust architectures, and anomaly detection. In parallel, privacy policies must be enforced to protect sensitive customer, employee, and partner data.
- Organizational Enablement and Data Literacy
A robust data strategy recognizes that technology alone is not sufficient—people and culture are equally critical. Employees at all levels must be empowered to use data in their roles. This involves investing in training, creating data steward roles, encouraging data-driven decision-making, and fostering collaboration between IT, operations, and business units.
- Agility and Innovation
In the face of rapid technological advances and market volatility, the data strategy should be agile and continuously evolving. It should support experimentation, pilot programs, and fast feedback loops. For instance, the use of AI in defect detection or supply chain simulation must be piloted, validated, and scaled quickly without bureaucratic delays.
- Sustainability and ESG Reporting
As companies face increasing pressure to meet sustainability goals, data strategies must include mechanisms to track environmental, social, and governance (ESG) metrics. This includes carbon footprint analysis, energy consumption optimization, and ethical sourcing transparency, all driven by accurate and timely data.
- Return on Data Investment
Finally, the company must treat data as a capital asset. This involves measuring and managing the ROI of data initiatives. Techniques such as data monetization, data-as-a-service (DaaS), or internal data marketplaces can unlock new revenue streams and justify ongoing investments in data infrastructure.
Data is no longer a byproduct—it is a critical resource for innovation and operational excellence. A well-defined data strategy that aligns with business goals, ensures governance, enables AI, and fosters a culture of data literacy is vital for sustaining growth in a competitive global market. By adhering to the criteria outlined above, manufacturing giants can transform data from a liability into a strategic advantage.